Drifting Away from the Mainstream
Media Attention and the Politics of Hyperpartisan News Websites
1 Introduction
Partisan media have grown in popularity in both Europe and the US. For example, the Reuters Institute’s Digital News Report 2020 indicates that 23 % of panelists in their study visited at least one partisan news website, such as Breitbart or The Blaze, each week. The report also documents the link between political partisanship and trust in public broadcasters on the part of those who identify with more left- or right-wing political views: in the context of an overall decline in trust in information from the BBC across all surveyed panelists in the UK, the biggest drop (20 %) is observed among people who consider themselves to be “very left- or right-wing” (Newman et al., 2020). Notably, countries with more polarized-pluralist media systems, such as Italy and Spain, have also been found to have lower level of trust in media and more populist communication (Humprecht et al., 2020).
These changes make it important to examine the increasing popularity of hyperpartisan news media, particularly among left- and right-wing populists in Europe and the US. This paper is part of a wider study of populism and online media and links populist support with the audiences, content, and networks of hyperpartisan media outlets. We combine datasets from multi-country surveys and website visits datasets in one of the first comparative studies of hyperpartisan news media that examines audiences, news domains, and content.
Our study has been organized as follows. First, we answer the question of who visits hyperpartisan media sites by linking website visits to panelists’ political affiliations and their trust in media. Then, we explore the global and national network of hyperpartisan media domains by measuring their audience similarity using the web-tracking dataset. Finally, we conduct an in-depth analysis of the content of hyperpartisan websites by combining qualitative and quantitative linguistic analysis of the news articles on pages visited by panelists at hyperpartisan news domains. Findings from this research provide insights into the ecology of hyperpartisan media, media trust, populism, and the rapidly changing online media landscapes of the US, the UK, Germany, France, Spain, and Italy.
We use quotation marks for “news” from hyperpartisan sites because, according to our definition, this material often falls outside of the – no doubt idealized – criteria for newsworthiness in democracies. As Jungherr and Schroeder (2022) have argued, it remains important to distinguish between those (often) traditional media that adhere to ideals of newsworthiness and journalistic norms and hyperpartisan and other online “news” sources that do not (we will return to this shortly). Here, we define hyperpartisan websites in a neutral manner, with hyperpartisan taken to refer to news or political information that falls outside of objective, professional, impartial, independent, diverse, and inclusive media. That is, we define hyperpartisan media in contrast to traditional media, including digital forms of legacy media, which have been a core characteristic of democracies and which uphold, however imperfectly, journalistic norms, thus importantly playing a gatekeeping role. Hyperpartisan “news” websites also push a narrow rather than broad range of topics – in terms of coverage of societal interests – because they do not have to be diverse and inclusive, with some material on hyperpartisan websites not even meeting the newsworthiness criterion in terms of either structure or content. We will return to this definition after reviewing related work.
2 Literature Review
Extant empirical research suggests the social impact of traditional and digital media in terms of influencing political behaviors (Robertson et al., 2018). Although various terms related to “hyperpartisan” websites deserve brief discussion, we shall focus here on recent contributions rather than providing a comprehensive summary of this fast-growing literature, most of which has focused on disinformation (see Bennett & Livingston, 2020). In relation to alternative “news” media, we do not define hyperpartisan media in contrast to hegemonic news media, as do Holt et al. (2019). However, Holt et al. do correctly note that “alternative media” has historically mainly been used in the context of media that champion progressive or left-wing causes, bypassing traditional channels. Meanwhile, reflecting changing trends in the digital news industry, the Reuters Digital News Report uses “alternative or partisan websites,” defined as “websites or blogs with a political or ideological agenda with a user base that tends to share these often-partisan views” (Newman et al., 2018, p. 45). Our definition partly overlaps with this because we are concerned with populism and leave open the question of whether populists are partisan per se. Furthermore, there are clearly political positions other than populism that can be considered partisan, and news media can be partisan in the sense that they have political leanings, as noted by Hallin and Mancini (2004). However, these alignments are typically with party positions that are made explicit and are well-established, as opposed to the more novel phenomenon of hyperpartisan media positioning themselves against what they consider the defects in traditional media and challenging existing parties.
Thus, our definition builds partly on the self-descriptions of “hyperpartisan” websites, which focus on offering a counterpoint to “mainstream” news or information sources. However, our classification also concerns the features that hyperpartisan sources exhibit or lack. Hyperpartisan websites do not appeal to broad audiences or cover a diverse range of topics. Instead, they cater exclusively to certain audiences, focus on certain issues, and adopt strong views on those issues, unlike traditional news media. However, we do not intend to predetermine whether this means that their “news” is not newsworthy from a perspective that regards gatekept news as not catering to those strong views. Another term that has been used is “junk news,” employed in several reports (see Howard, 2020) investigating computational propaganda with the main aim of identifying the source and spread of deliberate propaganda or lies. Although Benkler et al. (2018) also use “hyperpartisan,” they fail to provide a clear definition that sets it apart from “propaganda,” a term they also employ and which clearly falls outside of gatekept news in terms of journalistic norms. Thus, we avoid a focus on disinformation or misinformation. These deliberate and underhanded ways of spreading falsehoods fall outside our purview, although the degree to which non-gatekept information should be kept to a standard of journalistic accuracy, reliability, or objectivity remains an important question (Jungherr & Schroeder, 2021).
Thus, “hyperpartisan” here refers to news or political information that adopts a fringe or challenger political position not in contrast to the political “mainstream” but rather as a departure from the current democratic norms according to which media are autonomous and abide by scientific norms (i.e., knowledge should be reliable and evidence-based) and journalistic norms (i.e., objectivity, impartiality, inclusiveness, and diversity) (Jungherr & Schroeder, 2022). Although these norms might seem to exclude populist politics, that does not follow because populists could adhere to these norms while remaining populist (in their anti-elitism, for example, if, for example, elites are not scientific, or the media, if media elites are not impartial). We shall revisit this point in our conclusion, having assessed how hyperpartisan content and form fall within and outside our criterion. Finally, we must add that our data collection phase precedes the COVID-19 pandemic, meaning the topic of scientific disinformation falls outside this study’s scope (but see Jungherr & Schroeder, 2021).
2.1 Hyperpartisan Media and Social Media
Although this paper’s focus is identifying direct access to hyperpartisan content (web clicks), a different but related approach has involved gauging the extent to which hyperpartisan news or user-generated hyperpartisan content is shared on social media (Benkler et al., 2017; Barnidge and Peacock, 2019; Mourao and Robertson, 2019). Xu et al. (2020) investigated the sharing of hyperpartisan news on Facebook for the US, examining source transparency, content styles, and moral framing in relation to the issue of immigration. Sharing news, which indicates a high level of engagement, provides a useful complement to our study of the reach of hyperpartisan sites. Bhatt et al. (2018) studied the consumers of and web traffic to hyperpartisan news websites based on 668 hyperpartisan news domains and their Facebook pages (dataset shared by Buzzfeed were coded as right- or left-leaning), revealing that almost one third of hyperpartisan websites, especially right-leaning examples, were created in 2016 before and during the 2016 US presidential election. Strikingly, 11 % of hyperpartisan websites were found to be operated from Macedonia. More generally, sharing and obtaining news has been affected by social media and search engine platforms (Nielsen & Ganter, 2022), shifting the media environment by assigning a greater role and gatekeeping function to the likes of Facebook and Google, which have changed how news content is accessed.
Furthermore, a cross-country survey of populist attitudes and media use has suggested a link between populism and media preferences, with survey participants who hold populist attitudes less likely to consume news from quality newspapers (Schulz, 2018; Schulz, 2019). However, according to a survey-based study (Müller & Schulz, 2019) considering the case of Alternative for Germany (AfD), those with populist attitudes were neither more nor less likely to be exposed to public sector media and nor were they less educated. Furthermore, in another study comparing several countries by Schulz (2018) she showed that populists were in fact more likely to be exposed to public service news. These puzzling findings prompt our research question about the kinds of political support that correlate with hyperpartisan news exposure, a question we tackle comparatively.
Research Question 1 (RQ1)
Populists claim that traditional news media do not adequately represent them and challenge how they are represented in the media. Hence, building on previous research, we arrive at our first research question: What are the audiences for hyperpartisan websites? Extant findings link political attitudes with social media use and the consumption of hyperpartisan media. This can be explored by testing the following hypotheses, with H1–H 3 centered around factors for visits to hyperpartisan media and H4–H6 focused on the average attention paid to hyperpartisan media:
Hypothesis 1 (H1 ). Support for right-wing populist or left-wing populist parties is positively associated with visits to hyperpartisan media websites.
Hypothesis 2 (H2 ). Visits to hyperpartisan media websites are positively associated with level of political interest.
Hypothesis 3 (H3 ). Users with a higher level of trust in social media platforms and a lower level of trust in public broadcasting media are more likely to visit hyperpartisan media websites.
Hypothesis 4 (H4 ). Support for right-wing populist or left-wing populist parties is positively associated with the average attention paid to hyperpartisan media websites.
Hypothesis 5 (H5 ). Average attention paid to hyperpartisan media websites is positively associated with level of political interest.
Hypothesis 6 (H6 ). Users with a higher level of trust in social media platforms and a lower level of trust in public broadcasting media are more likely to spend longer time online.
2.2 Populism and Alternative Media
There has been extensive research on the relationship between populism or the radical right and alternative media. Müller and Schulz (2021) investigated alternative media use during the period of the 2017 German election, focusing on alternative media with an affinity to populism (AMP). Their findings, among other things, “suggest that also among truly populist AMP frequent users, education and quality newspaper usage are higher than average” (2021, p. 288). A study by Müller and Freudenthaler undertook topic modeling of the content of several German-language right-wing alternative media and demonstrated that “populist notions are indeed central to far-right alternative news media in Germany” (2022, p. 20). The larger picture is that populists challenge conventional media, claiming that they distort the news and neglect the political positions of what they regard as the “true people,” which keeps pace with populist politics and its anti-elite stance and pro-exclusionary conception of “the people” (Jungherr et al., 2019). Hyperpartisan media, to which we can now turn, allow them to do so.
Alternative Media or Hyperpartisan Media?
Rae (2021) has argued for “hyperpartisan” rather than “alternative” media or news: both differ from traditional news media, but “alternative” refers more to the self-image of the alternative right or left, whereby they claim to provide political options outside of the “mainstream.” “Hyperpartisan,” Rae says, applies more to populist media that are “transgressive in style” and claim to be news while forsaking journalistic norms of objectivity with “attacks [on] the other side’s point of view” (2021, p. 1,118; see also Holt, 2018), mirroring the populist idea of “the people” versus “elites” or “us” versus “them.” Rae also argues that these outlets engage in personalization or bias towards leaders. However, although that may be true of populist contexts with majoritarian political systems (e.g., the US), it does not necessarily reflect the situation of European populist parties, which can be more policy- or party-centric rather than leader-centric (the German AfD and the Sweden Democrats are good examples; see Gerbaudo, 2018, and Moffitt, 2017). However, hyperpartisan sites lack balance and a diversity of perspectives, especially among populists (Waisbord, 2018). Thus, Rae’s approach is useful because she argues that identifying particular digital platforms as hyperpartisan provides a starting point for understanding the anti-media and anti-elite agendas of populists.
Hyperpartisan Media and Populism
We employ Mudde’s (2007) well-established definition of populism, which comprises three elements: It is anti-elite, people-centric, and promotes additional power for “the people.” To this, we add exclusionism towards a group or a set of forces apart from the elite, typically immigrants in the case of right-wing populism and welfare chauvinism or resistance to foreign globalizing economic elites in the case of left-wing populism. The literature on support for populist parties and populist attitudes has grown quickly in recent years (see, for example, Van Hauwaert & Van Kessel, 2018; Norris & Inglehart, 2019), and there have been several debates related to populists and media (de Vreese et al., 2018; Moffitt, 2017; Krämer, 2018). Based on a representative telephone survey of German citizens, Fawzi (2019) found that “citizens who are skeptical about the political elite clearly transfer this negative evaluation to the media.” (p.158) This is because they perceive the media to belong to the political elite: Media and politics are seen as part of one conglomerate representing the same establishment, a view that also holds for journalists from tabloid media. Jungherr and Schroeder (2022) have discussed more generally how, in Germany and the US, for example, populist criticisms of the media and their online alternative or partisan news sources are in tension with journalistic norms and the functions of the public sphere (or arena).
The study of non-traditional news websites, particularly on the right, has seen several different approaches. Heft et al. (2020) studied 70 right-wing online news sites in Europe and the US to gauge their online visibility using Alexa and social media followership. They also considered the volume of content presented and coded aspects of this content. They observed considerable variation between the countries studied, with some right-wing online news having an unexpectedly stronger user base (Sweden, Germany, and the US) and others a weaker one (Denmark, Austria, and the UK), and revealed a mixture of characteristics of news sites (for example, the transparency of organizations and their funding strategies). A different approach to measuring the prominence and reach of these types of online news sites involves measuring their transnational connectedness and their location within wider networks. This is what the same authors did for a larger (150) set of news sites (Heft et al., 2021) in the same set of countries, revealing, for example, that Swedish and German sites were most nationally linked, while Danish ones were most transnationally linked. The US was highly integrated nationally and served as a transnational hub. These studies indicate the important variety of these news sites, with the limitation that they cannot link users with different political attitudes to particular website uses. We will consider how our findings correspond to theirs in the conclusion.
As mentioned, hyperpartisan websites can also be seen as part of a separate set of debates about mis- or disinformation and propaganda, including bots and information warfare. Another recent debate specifically relating to populism has concerned their increase in attacks on media, which populists sometimes label “fake news,” attacks particularly concentrated on public service media and so-called “mainstream” media (see Sehl et al., 2020). This fits with our definition insofar as populists are not satisfied with gatekept media.
Research Question 2 (RQ2) and Research Question 3 (RQ3)
The extant empirical research has analyzed the relationship between alternative media and hyperpartisan media and examined the content of hyperpartisan media. However, how hyperpartisan media websites are intertwined and how media agendas regarding hyperpartisan media vary between different countries remain under-explored. Therefore, we ask, in (RQ2), to what extent do hyperpartisan websites comprise a network of political information, as measured by their audience similarity? Finally, drawing on findings about the variations in content and outlets between different countries, we examine, in (RQ3), what is the news content of hyperpartisan media?
Our questions seek to establish whether, for example, populist audiences for news and political information are more prone to depart from mainstream news in what they read and watch in the sense that the broadly accepted common ground or the rules of the game in democracies for what is considered journalism or newsworthiness do not apply. Examples include denying rights of political participation to legitimate groups and not accepting a fact-based basis for discussion. This also points to the distinction made in the previous section: “alternative” refers to an alternative to news media and to journalistic norms, no matter the political orientation, an alternative that lies outside of the norms of political impartiality and professional journalism of traditional media.
3 Methods
We have combined surveys and web tracking to study the users, networks, and content of hyperpartisan media. Using a mixed methods design, this paper addresses three fundamental research questions related to hyperpartisan media: What are the audiences for hyperpartisan websites? To what extent do hyperpartisan websites comprise a network of political information, as measured by their audience similarity? what is the news content of hyperpartisan media? Figure 1 visualizes this study’s workflow. To answer these questions, we first conducted regression analyses of the survey responses regarding the use of and attention to hyperpartisan online media news platforms, with trust in media, political attitudes, and demographic variables employed as independent variables. Second, we explored the networks of hyperpartisan media based on web-tracking data. Third, we analyzed the content of hyperpartisan media websites using qualitative and quantitative approaches. Combining these methods has allowed us to conduct a multi-dimensional study of hyperpartisan media in the UK, the US, and four European countries, namely, France, Germany, Italy, and Spain.
Figure 1: Workflow of this paper
3.1 Data Collection
Our study is a unique combination of an online user behavior dataset, collected from desktop Internet logs, and a large-scale attitudinal dataset built upon survey responses to various questions related to political opinions and demographic variables. The data collection of web tracking and survey data for this study was performed by the survey company Netquest (an affiliate of GfK, the largest German market research company) in full compliance with the EU General Data Protection Regulation laws (Yan et al., 2021).
The data used in this study were collected in France, Germany, Italy, Spain, the UK, and the US between February and June 2019. A total of 9,625 participants responded to the survey study concerning media trust, political attitudes, populist attitudes, and demographic variables. The web-tracking dataset includes over 150 million website visits from 7,230 panelists in the six countries. It should be noted that although we collected web-tracking data from both desktop and mobile devices, we have only used the desktop web-tracking data because mobile web-tracking data only shows visits to mobile applications rather than specific URLs, presenting challenges for exploring users’ everyday consumption of political content. The web-tracking dataset based on desktop devices included both news and non-news website visits. Our research team ranked all website domains by their popularity, measured by numbers of total visits, and systematically coded the 5,000 most-visited domains per country into political and non-political domains. Because the distribution of public attention online is unevenly centered on top-ranked domains, there is a long tail of rarely visited websites. Having identified the political websites in the first round of coding, we then coded political websites into six categories: legacy press, tabloid press, commercial broadcasters, public broadcasters, digital-born outlets, and hyperpartisan news. “Hyperpartisan” was coded as “news sites promot[ing] a narrow and skewed political agenda without making an effort towards a balanced representation of major political issues, events[,] or political actors” (see Stier et al, 2020 for all codes and Yan & Schroeder, 2021 for climate news agenda analysis). Consensus from two independent coders enabled us to arrive at these categorizations.
Figure A1 in Supplementary Material A shows the percentage of users who visited different types of news domains in each country, with the percentage of visits to hyperpartisan news domains highlighted in green. Hyperpartisan news websites reach the highest percentage of audience in Spain and the US, with 11.19 % and 6.83 % of panelists who participated in the web-tracking study visiting hyperpartisan media at least once. Figure A2 in Supplementary Material A shows the number of distinct visitors and the average political orientation scores of hyperpartisan news domains. Political orientations of hyperpartisan news websites are measured by aggregating the political orientations of panelists who visited these websites, drawing upon the survey data. The figure suggests a more right-leaning political stance of hyperpartisan news websites than the average political orientations of news websites (more than 7 compared to around 6), validating the coding of hyperpartisan media by our research team.
To check the validation of our coding of hyperpartisan media, we examined the media slant scores of the hyperpartisan domains by calculating political slant of all news domains based on average scores of political orientations of visitors who have consumed the domain. Figure A 2 shows the distribution of media slant scores of hyperpartisan domains, which are compared to the media slant scores of other non-hyperpartisan domains. The visualisation provides face validity to our coding of hyperpartisan media, showing that hyperpartisan media have more left- or right-leaning media slants than other media outlets. In Figure A 3, we can see that non-hyper partisan media have a more moderate audience.
3.2 Measuring Media Attention to Hyperpartisan Media
Our first research question was designed to understand who is more likely to consume and pay attention to hyperpartisan media websites and what political and demographic factors are associated with the use of (and time spent on) hyperpartisan media websites. To address these questions, we use variables in the cross-country survey and created dependent variables based on a web-tracking dataset. The supplementary material provides survey questions for all variables used in the regression analysis, as well as descriptive statistics. The survey dataset and panelists’ web-tracking dataset are matched by anonymized panelist IDs, with participants who opted in for only one of the studies (either the web-tracking or survey study) omitted from the regression analysis.
Dependent Variables: Visits and Attention to Hyperpartisan Media
To categorize the websites in the web tracking dataset, we first coded the top five thousand most visited domains in each country into political and non-political websites, yielding 574 distinct political website domains in six countries. Then, these politically relevant websites were categorized into five types by three researchers with domain expertise: quality print outlets (including magazines), tabloid press, commercial broadcasters, public broadcasters, digital-born outlets, and hyperpartisan news (where “news” includes all political content) (for the coding procedure, see Stier et al., 2020). In this paper, we are interested in exploring the consumption of and attention to hyperpartisan media in the UK, the US, France, Germany, Spain, and Italy.
We created two dependent variables based on news website visits: 1) Hyperpartisan media visit: a dichotomous variable indicating whether or not a panelist has read news articles from hyperpartisan news domains; 2) Hyperpartisan media attention: a continuous variable that measures the attention that a panelist has paid to hyperpartisan news, measured by the average length of time (in seconds) that panelists spent viewing news articles from hyperpartisan websites, calculated as:
Hyperpartisan media attention =
Independent Variables:
Media Trust, Political Attitudes, and Demographics
We used three groups of independent variables based on cross-country surveys to identify which factors are associated with visits and attention to hyperpartisan news online:
1)Political attitudes. Support for right- and left-wing populist parties (or leaders in the US)1, political orientation, political interest, and belief in political efficacy.
2)Media trust. Degree of trust in different digital media, including social media platforms, public broadcasters, private broadcasters, and newspapers.
3)Demographic variables. Socioeconomic status of panelists as measured by level of education and income and demographic variables including age, gender, and metropolitan versus non-metropolitan status. We also created a country dummy variable to control for country variances in the model.
We also controlled country variances by adding a categorical variable of panelist’s country of residence in the regression model. France is the reference category in the country variable.
3.3 Constructing Networks of Hyperpartisan Media
Previous research has constructed the networks of news websites by defining edges as the shared audience between them by mapping the shape of website visits through community detection. For example, Wu and Taneja (2016) visualized the structure of website visits via a network analysis of world Internet traffic data. The authors measured shared audience using “audience duplication,” which consists of the proportion of any pair of websites being visited by the same cohort of users within a given time period. They also introduced a random duplication measure that considers an audience duplication value if it is exceeds the expected duplication of any pair of websites resulting from chance. Another group of scholars has criticized the lack of statistical testing of observed overlaps in this method and instead proposed mapping the audience network of news media websites by analyzing the backbone network of websites (Majó-Vázquez et al., 2018). This method only keeps overlapping ties between any pair of websites that are statistically significant at the threshold of p <.05, which rejects the null hypothesis that the observed overlap is due to a random overlap between websites. Despite the differences between these two groups of researchers in terms of filtering the edges between any pair of websites to construct the audience network, both approaches measured the likelihood that any pair of websites is shared/visited by the same group of users.
This paper proposes a different approach to constructing the network of news websites by applying a cosine similarity algorithm to calculate the similarity between any pair of websites. The cosine similarity between each pair of websites consists of the value of edges in a weighted network of news websites. This approach has been commonly applied in the Internet industry to understand the similarities of Internet content or purchased items between user groups or the similarities of audiences or consumers between different websites or products.
To apply this method, we first generated a matrix with a binary attribute of 0 and 1 based on the raw dataset of the web website visit dataset, with each column in the matrix representing a news website and each observation/row indicating the news website visit history of a panelist. In the binary matrix, one means the panelist has visited a website at least once. We only include website visit sessions that last longer than 0 seconds. Zero means the user has never visited a given website. In the next step, we calculated the similarity score of all pairs of news websites (N = 574). We applied cosine similarity to project the network of panelists and news website visits into a network of news websites, with edges measured by the cosine similarity between any pair of websites. Cosine similarity considers the attributes (visits to distinct websites) of any points (panelists) within a dimensional space and uses cosine distance to measure angular distance. Thus, the cosine similarity of websites A and B is equal to the cosine of the angle between two attribute vectors XA and XB. The cosine similarity between two websites that have the exact same visitors is always equal to 1.
cosine similarity =
After processing and calculating the similarity network in Python using networkx, we could arrive at a weighted network, with nodes representing 574 distinct news websites and 57,399 edges representing the cosine distance between any pair of websites (with edges that have a score of 0 filtered out). A similarity matrix of news domains was calculated in Python. Then, we exported the matrix that contains the cosine similarity scores of all pairs of websites into Gephi to conduct community detection, visualize the network, and calculate network statistics.
In the analysis of network characteristics, we used two measurements of network features: PageRank and an E-I index. Page rank is among the most used measurements of node centrality in network analysis. The underlying assumption is that the more central a node, the higher the quantity and the better the quality of other pages that link to that node. PageRank scores of nodes in the audience similarity network were calculated in Gephi. We also used the E-I index to measure how global or local a news domain is in the audience similarity network. The E-I index was first introduced by Krackhardt and Stern (1988) to calculate the homophily index that measures the extent to which a node is connected with nodes external to the node’s own partition. The index is calculated as follows:
E-I Index =
where EL=the number of external links;IL=the number of internal links
In our analysis, because all edges in the network are weighted, we modified the calculation of the E-I Index to have EL = the sum of weights of all external links and IL = the sum of weights of all internal links. The EI index value ranges from -1 to +1, where +1 indicates all links to the node are external to the node’s partition, and -1 means all links to the node are internal to the sub-group. Our paper’s community detection algorithm identified that news websites are clustered based on linguistic differences. Thus, the E-I index in this paper measures the extent to which a node or a group of nodes establishes cross-linguistic connections: The higher the E-I index, the more globally connected the news domain in the audience similarity network.
Content Crawling and Text Cleaning of Hyperpartisan
Media News Titles
Text Crawling. We used two Python web crawlers, DiffBot and Newspaper,2 to scrape content from all hyperpartisan media URLs in our news website visit datasets. Among the 18,544 distinct URLs from hyperpartisan media news domains, we successfully collected 16,568 articles. Although some URLs were no longer valid, we found that their URLs contained article titles.3 We use regex expression in Python to extract 1,619 further titles of hyperpartisan news articles, producing a total of 18,190 titles of hyperpartisan media website articles. The successful content crawling/extracting rate was 98.09 %, slightly undermined by issues such as URLs no longer being available or news websites requiring paid subscriptions.
For content analysis, we removed 64 URLs that are home pages or index pages of hyperpartisan news domains.4 Because these pages are constantly refreshed, content often differs from the time that our project’s panelists participated in the web-tracking study. We also removed 678 news articles published in 2020, which is outside our project’s period of data collection.
Text Translation and Cleaning. We used the Baidu Translator API5 to translate all non-English titles. Researchers in this field have recommended translating entire documents rather than the document-term matrix (DTM) when using machine translation to prepare topic modeling (Reber, 2018). English articles account for almost 50% of the text dataset. We provide a summary table of titles in different languages in Supplementary Material C (see Table C1). We qualitatively accessed a sample of machine translation results and found that the accuracy of machine translation is acceptable, with the main exceptions being misunderstanding or not recognizing names (for example, of politicians) or missing linguistic nuances. After machine translation, 17,448 titles of hyperpartisan news articles and 187,993 English words remained for content analysis.
All translated titles were tokenized into comma-separated tokens after cleaning punctuation and replacing capitalized characters with lower-case characters. We used the NLTK library in Python to remove common English stop-words. To identify combinations of frequently co-located expressions, we applied bigram models using the gensim library to find word combinations that appear together at least ten times in the corpus. All words were lemmatized into the lemma forms of words using the spacy library. Then, we applied part-of-speech (PoS) tagging on all words. The final corpus of hyperpartisan news media titles comprised only nouns, verbs, and adjectives.
Keywords Extraction and Topic Modeling. After text cleaning, the corpus of hyperpartisan news titles comprised a total of 114,454 words. To extract and visualize keywords in hyperpartisan news websites, we first used term frequency-inverse document frequency (TF-IDF) to measure the importance of keywords within each hyperpartisan document. This measurement considers the fact that, in natural language, some words appear more frequently, regardless of their semantic importance in documents (Piantadosi, 2014). Compared to word frequencies (TF), which measures the absolute number of occurrences of words, TF-IDF scores show the relative importance of words in a collection of documents.
In this paper, we used topic modeling, an unsupervised machine learning approach, to identify latent semantic topics in hyperpartisan news articles. Among the commonly used topic modeling methods is the Latent Dirichlet Allocation (LDA) model proposed by Blei et al. (Blei et al., 2003). LDA topic modeling is based on two latent topical distributions: the word-topic matrix and the document-topic matrix. Although LDA is an unsupervised learning approach to identify latent topics in a corpus, it is important for researchers to set the optimal parameters, such as the number of topics, k. To choose the optimal k for the topic model, we used the topic coherence measurement, which calculates the levels of semantic similarity between topics to select those topics that are semantically meaningful (Evans, 2014). We set the number of topics equal to 11, the number at which the topic coherence score reached the second highest coherence value in this case and where keywords concerning different topics do not have too much repetition (see Figure C1 for the value of coherence scores at different k values). We also validate the topic modeling result by qualitatively interpreting the content of the identified topics as well as visualizing topics using LDAvis6 (internal validation) and check whether the geographical patterns of certain topics corresponded to the location of political events that happened during the data collection period (such as the Mueller investigation) (Maier et al., 2018). We used the gensim library in Python to train the LDA model. When we trained the LDA model, the random state of the model was set to 100 (random_state = 100), which is useful for the model’s reproducibility.
4 Findings
4.1 Who Consumes Hyperpartisan Media?
We provide a table with summary statistics of models for the consumption of (logistic regression) and attention to hyperpartisan media websites (multiple linear regression) in the six countries of interest, with political attitudes, media trust, and demographic variables as factors in the models (see Table B1 in the Supplementary Material for descriptive statistics and question wordings of the independent variables; see Table B2 for regression results on models of visits and attention to hyperpartisan media websites). We find that political attitudes and media trust variables are both significant factors accounting for whether a panelist has visited a hyperpartisan website. Figures 2 and 3 provide visualizations of the odds ratio and coefficients of the two models.
Compared to non-supporters of populist parties, the odds of right-wing populist party supporters visiting hyperpartisan media websites is 1.421 times higher than the odds for supporters of non-populist parties or leaders (B = .351, p < .01). Left-wing populist party supporters are also more likely than other voters to visit hyperpartisan media websites, but the difference is only significant at the 90 % confidence interval (B = .213, p < .1). Panelists who are interested in political issues also record higher odds of visiting hyperpartisan websites (B = .279, p < .001). We also find that panelists with higher levels of trust in social media (B = .134, p < .01) and lower levels of trust in public broadcasters (B = -.192, p < .001) are more likely to consume hyperpartisan media. This finding supports insights from previous empirical research linking the declining trust in public media (Holt et al., 2019) and increasing reliance on social media platforms (Lewis, 2018) with the use of alternative news sources, such as hyperpartisan media. Therefore, based on the regression results, we partially accept H1 , with a link identified between right-wing populist parties and visits to hyperpartisan media websites, but no link identified between left-wing populist parties and visits to hyperpartisan media websites. Our findings also support H2 and H3 , with higher levels of interest in politics, trust in social media, and distrust of public broadcasters positively correlated with the consumption of hyperpartisan news.
One surprising finding about the link between education and the consumption of hyperpartisan media websites is that people with intermediate (B = .276, p < .05) and high levels of education (B = .315, p < .05) are more likely to visit hyperpartisan media websites than those with a low level of education. However, we find that panelists in the intermediate- (B = -.331, p < .001) and high-income (B = -.431, p < .001) groups are less likely than people from the low-income group to visit hyperpartisan media websites. Differences also exist for gender and age, with females and younger-generation panelists less likely than males and the older generation to visit hyperpartisan media websites.
Figure 2: Visualization of the odds ratios of the regression model for visits to hyperpartisan media websites. The odds ratios and confidence intervals are represented by parallel lines. Red labels indicate a negative association between the variable and visits to hyperpartisan websites, while blue labels indicate a positive association between the variable and visits to hyperpartisan websites. Country odds ratios are in comparison to France
Figure 3: Visualization of the odds ratios of the regression model for average attention to hyperpartisan media websites. The odds ratios and confidence intervals are represented by parallel lines. Red labels indicate a negative association between the variable and attention to hyperpartisan websites, while blue labels indicate a positive association between the variable and attention to hyperpartisan websites. Country odds ratios are in comparison to France.
Having noticed the country variances in hyperpartisan media visits and the empirical evidence concerning the links between right-wing populist party support and visits to hyperpartisan media and between media trust and visits to hyperpartisan media, we present plots representing interactions between right-wing populist party support and country, social media trust, and public broadcast trust in Figures 4, 5, and 6 (Supplementary Material B includes the regression tables featuring the interaction terms). The two-way interaction between our key independent variable (i.e., right-wing populist party support) and the variables of country and media trust reveal the effects of interactions between two independent variables. Table B3 shows that the interaction term between right-wing populist party support and the US is statistically significant (p < .05), with the pseudo-R2 bigger with the interaction term than in the model without it (0.1382 vs. 0.1369). This indicates an interaction effect between support for right-wing populist parties/leaders and the US. This finding aligns with the country variations in media systems described by Hallin and Mancini (2004): We found that hyperpartisan media plays a more important role for right-wing populists in the US. Figure 5 shows the country variances in right-wing populist party support in predicting the likelihood of visiting hyperpartisan media websites. Here, the line for the US is steeper than the lines for other countries, which suggests a possible interaction effect based on country and right-wing populist party support. That is, supporters of right-wing populist parties/leaders in the US (i.e., supporters of Trump) are more likely to visit hyperpartisan media websites.
Inspired by Lewis’s research (2018) on the alternative media ecology of social media, we are interested in further exploring the interactions between social media trust, populist party support, and visits to hyperpartisan media. The differences between supporters and non-supporters of right-wing populist parties in terms of visiting hyperpartisan media websites is reinforced when the panelists have less trust in public broadcasters (Figure 5) or more trust in social media (Figure 6). However, the interactions between right-wing populist party support and trust in public broadcasters or trust in social media are significant at either the 90 % confidence level or not statistically significant (Tables B4 and B5 present the interaction terms between right-wing populist party support and trust in public broadcasters and social media).
Figure 4: Interaction plot of country and support for right-wing populist parties in the logistic regression model of visits to hyperpartisan media websites
Figure 5: Interaction plot of trust in public broadcasters and support for right-wing populist parties in the logistic regression model of visits to hyperpartisan media websites
Figure 6: Interaction plot of trust in social media and support for right-wing populist parties in the logistic regression model of visits to hyperpartisan media websites
Neither the political nor the media trust variables significantly explain variances in the average length of time a visitor spent reading hyperpartisan media news articles (see Figure 3 for a visualization of the regression model). Therefore, we reject H4 , H5 , and H6 . Thus, although whether or not an individual visits hyperpartisan media websites is associated with political factors, the amount of attention given to hyperpartisan content varies across non-political factors, including news content characteristics and website design.
Notably, older-generation panelists (B = -.869, p < .001) are more likely than younger-generation panelists to consume more time-consuming political information on hyperpartisan media websites.
4.2 The Audience Similarity Network of Hyperpartisan Media
We conducted community detection, based on the modularity algorithm, on the entire audience similarity network and (unsurprisingly) found media websites clustered by language. Thus, for news websites across the six countries of interest, we identified five linguistic communities, that is, English, Spanish, German, French, and Italian sub-networks. Nodes in the network represent different news website domains, and the weight of edges are the cosine similarity scores between each pair of news domains. Figure 7a visualizes the audience similarity network of hyperpartisan media website domains, which includes 50 nodes and 379 edges. As for other types of news domains, hyperpartisan media websites clustered by languages are divided into five linguistic communities of audience similarity networks. Nonetheless, some websites, such as dailykos.com and rt.com, traverse linguistic communities (Figure 7b) according to their audience similarity scores. We suggest that researchers interested in the qualitative analysis of hyperpartisan media websites use this measurement of audience similarity scores to identify websites with similar audience cohorts.
We were further interested in knowing whether the structural characteristics of hyperpartisan domains – such as PageRank and the E-I index – can inform researchers about hyperpartisan media sources that are structurally important in the audience network. Figure 8 shows the top 20 hyperpartisan news domains as ranked by PageRank, E-I Index, number of distinct panelists, and average duration of visit.7
Figure 7: Visualization of user similarity network of hyperpartisan media websites, with communities identified and colored by modularity scores (layout algorithm is Force Atlas 2; node sizes ranked by PageRank) and the top ten most similar pairs of hyperpartisan news websites.
(a)
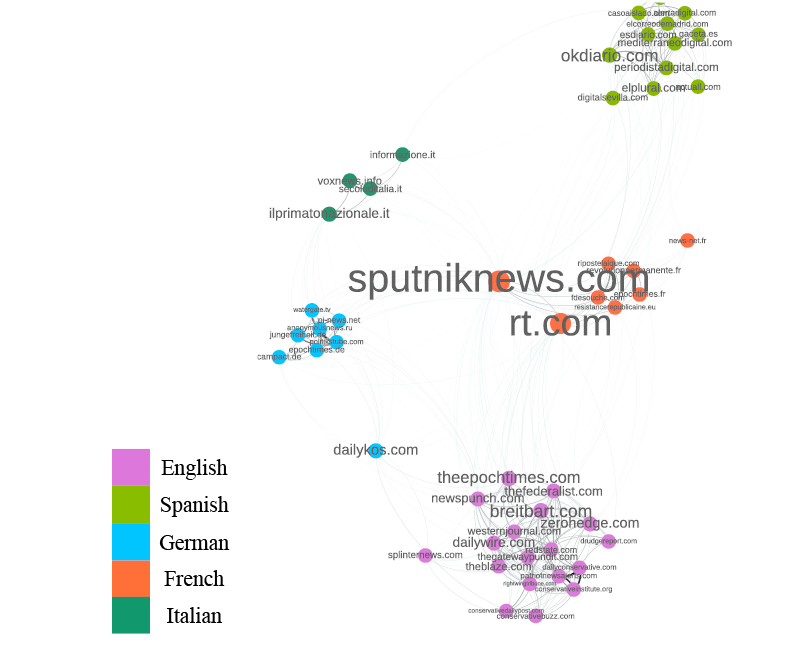
(b)
Top 10 pairs of most similar hyperpartisan news media websites |
|||
Rank |
Domain A |
Domain B |
Similarity |
1 |
dailyconservative.com |
patriotnewsalerts.com |
0.783 |
2 |
dailyconservative.com |
conservativeinstitute.org |
0.667 |
3 |
patriotnewsalerts.com |
conservativeinstitute.org |
0.639 |
4 |
politikstube.com |
anonymousnews.ru |
0.630 |
5 |
anonymousnews.ru |
watergate.tv |
0.471 |
6 |
politikstube.com |
jungefreiheit.de |
0.445 |
7 |
ripostelaique.com |
fdesouche.com |
0.429 |
8 |
thegatewaypundit.com |
rightwingtribune.com |
0.420 |
9 |
dailywire.com |
westernjournal.com |
0.407 |
10 |
politikstube.com |
watergate.tv |
0.401 |
Figure 8: Visualization of the E-I index, number of distinct panelists (using a logarithmic axis), average duration per visit to each hyperpartisan news media domain. Number of distinct panelists plotted in logarithmic scale with log base 10 to avoid overlaps of bubbles in the plot. Values for the number of distinct panelists in this plot could be interpreted as True value of distinct panellists= 10^(Observed value on x-axis).
Using network characteristics such as the E-I index can help researchers identify hyperpartisan media with a transnational audience. We visualized the number of panelists who visited hyperpartisan news domains and the reach (i.e., more global or more local) of those domains using the E-I index. Although some hyperpartisan media domains (e.g., rt.com and sputniknews.com) have transnational reach and are among the top-ranked domains in terms of the total number of panelists who visited the websites, several domains (e.g., politikstube.com) do not have a high total number of visitors but do have a high level of transnational reach when considering network characteristics. Another interesting finding is that some hyperpartisan websites (e.g., dailykos.com and splinternews.com), although not among the top-ranked domains by number of distinct panelists, record a high level of average attention per visit. These findings align with previous empirical evidence demonstrating that hyperpartisan media websites, as part of the information ecology of the Internet, are substantially divided on the basis of linguistic variances (Wu and Taneja, 2016; Taneja and Wu, 2019).
Notably, we identified some hyperpartisan media websites sitting at the crossroads of multiple countries, transcending national borders. Hence, our analysis of audience network demonstrates that web-tracking data could be used not only to understand the online media behaviors of individuals but also – when analyzed in an aggregated perspective via audience similarity scores – to suggest structural features of the global media infrastructure.
4.3 The Politics of Hyperpartisan Media
Figure A2 shows the inferred political orientation of hyperpartisan news domains based on the average political orientation scores of their visitors. The figure demonstrates that hyperpartisan media attract a rather extreme audience cohort: The average Left-Right political orientation for hyperpartisan media domains is 7.18, exceeding the average political orientation of all types of media domains (5.93), signaling the right-leaning stance of hyperpartisan media. Contrary to the Buzzfeed partisan websites dataset used by Bhatt et al. (2018), which coded 489 out of 668 partisan sites as left-leaning, we inferred the media political orientation of hyperpartisan media websites from their visitors and found that most of the hyperpartisan websites considered in our research were right-leaning.
For reasons of space, we qualitatively examine and compare the hyperpartisan media content for only two languages, namely, German and English, which correspond to countries with quite different media systems, based on Hallin and Mancini’s schema (2004). Germany features a strong public service orientation, with the US characterized by a strong commercial media orientation (among other differences). Meanwhile, although the two countries also feature different political landscapes, both ecosystems include populist support.
Given the dominance of English-language US-based content, German-language content provides an interesting comparison. On the left, there is campact.de, a left-wing/green progressive activist site modeled on moveon.org, a US-based domain. The website’s content calls for action on climate change, advocating for “Fridays for Future” and other campaigns and European-wide climate demonstrations. The same applies to the German version of the DailyKos site (which is among the English-language US left-wing hyperpartisan sites). The other hyperpartisan German-language sites are all pro-AfD right-wing populist sites (e.g., jungefreiheit.de and politikstube.com). These sites fall outside of the bounds of journalistic norms insofar as they present a tendentious and misleading version of the news. For example, a story on the Junge Freiheit page concerning immigration, a central topic on the site, feature the headline: “Left party: refugees should be able to choose their own host country.” This refers to the populist Left party (Die Linke) in Germany, and the story that suggests that this party favors an immigration policy in the coming EU election platform that would be harmfully generous to immigrants, which is misleading because the idea that refugees could choose their host country is not party policy. However, the story captures the anti-immigrant sentiments of the AfD, something similarly illustrated by a story that accuses the German government of keeping data concerning the number of refugees hidden from the public. Finally, a story on the Politikstube site about Greta Thunberg demonstrates the right-wing populist stance on climate change, suggesting that Thunberg is instigating a “class war” between the younger and older generations. However, it verges on conspiracy theory by suggesting that Thunberg has support from politicians and the “mainstream” media. This implies, especially in the video that accompanies the story, that there is a hidden global elite of celebrities backing the Swedish climate activist. Finally, there is also a story concerning a controversy about a well-known racist black trademark figure used in the packaging of chocolate sweets, which caused outrage over the so-called “political correctness” of removing this figure.
4.4 Extracting Keywords and Identifying Topics from Hyperpartisan Media Content
Having translated non-English hyperpartisan news titles into English and cleaned the corpora of hyperpartisan news titles, we computed TF-IDF, frequency-inverse document frequency, to extract keywords from the corpus of 17,488 hyperpartisan media article titles.
Figure 9 shows word clouds for the top-ranked 100 keywords in hyperpartisan titles in each language. Sizes of words in the word clouds represent TF-IDF scores. We found country differences in hyperpartisan media content. Hyperpartisan news websites in the US and the UK focused on US politics, especially Trump (and the Mueller investigation into Trump’s conduct). Meanwhile, hyperpartisan media in the European countries focused heavily on conflicts between religions (“Muslim”) and anti-immigration content (“refugee”). Nonetheless, each non-English corpus featured words related to US politics among the highly ranked keywords.
Figure 9: Word clouds for the 100 top-ranked keywords in hyperpartisan news article titles in each language. Word size indicates a word’s TF-IDF score
English |
Spanish |
German |
French |
Italian |
Using the top-ranked keywords for each topic, we assigned themes to 11 topics categorized in the topic model. We assigned the topic with the most weight in the document to the title (dominant topic) and calculated the percentage of documents featuring each topic as the most dominant topic (see Table 1 for a list of keywords in each topic). We also assigned topic themes to identified topics to increase the interpretability of the LDA findings.
Topics 5, 7, and 1 recorded the highest numbers of titles across all hyperpartisan media articles. Two out of three top-ranked topics related closely to US politics or directly to Trump’s administration (or the Mueller investigation of Trump’s conduct). US politics are covered in much detail in many articles. However, many keywords relate to female politicians. For example, Democratic politicians and pro-Democrat figures are mentioned in several articles, including house speaker Nancy Pelosi, judge Ruth Bader Ginsburg, and actress Alyssa Milano (e.g., “Alyssa Milano crashes and burns, mangles the truth in attempt to blast Trump over taxes”; “Newly resurfaced video highlights Nancy Pelosi’s glaring hypocrisy on the Mueller report”). Some titles are about women who are not celebrities, for example, “Woman who went viral and lost her job for harassing Trump supporter in Starbucks has gone missing.”
Topics 5, 2, and 1 are about the Mueller investigation and report, including keywords about the investigation such as “Mueller” and “Mueller report.” Although topics related to US politics were ranked as the most dominant topics in hyperpartisan news titles, there were also topics related to cultural or social issues, particularly in the European countries. For instance, Topic 3 includes issues related to social issues and immigration. Keywords related to both abortion and religious conflict after the “Notre Dame” fire appear in the context of this topic, with some hyperpartisan news articles spreading conspiracy theories about the link between the Notre Dame fire and the Muslim community (e.g., “Muslims claim to have burned Notre Dame in revenge for Christchurch”), highlighting the tension between the Christian and Muslim communities in France.
Another example is Topic 0, which includes articles related to local or national social news and accidents, with keywords such as “survivor,” “die,” and “dog” included in this topic. However, hyperpartisan media closely follow news related to political movements that do not relate to party policies, especially climate politics. Topic 8 includes climate change along with other left-wing political causes. For example, some articles concern the debate between climate activists and climate skeptics (e.g., “Bill Nye the Science Guy goes OFF about climate change”) and how Democratic politicians have discussed climate change: “AOC claimed world will end in 12 years if climate change not addressed. Now she’s changing her tune.” Political “protests” organized by left-leaning voters are also covered by hyperpartisan media outlets (e.g., “Far-left activists planning protest at home of Fox News’ Tucker Carlson”). Despite the appearance of some social issues in the identified topics (e.g., abortion, climate, accidents), most topics in hyperpartisan media articles appear to be politically relevant, especially to US politics: Figure 10 shows that the topics with the highest and third-highest proportion of hyperpartisan news articles in the US relate to Mueller investigation (i.e., Topics 5 and 7). The topic of the Mueller investigation of Trump also receives the most hyperpartisan media attention from Spanish and German hyperpartisan websites.
Table 1 Topics identified by LDA topic modeling and top-ranked keywords included under each topic
Topic Theme |
Index |
Keywords |
# Dominant Topic |
Prop |
Mean |
Mean |
Mueller |
5 |
trump, sign, petition, democrat, comment, send, congress, mueller, omar, house |
2654 |
0.152 |
0.502 |
1.795 |
Elections |
7 |
campaign_action, trump, woman, say, go, face, watch, day, |
2256 |
0.13 |
0.522 |
1.866 |
Mueller |
1 |
report, trump, pelosi, mueller, watch, boy, border, fbi, find, end |
1860 |
0.107 |
0.527 |
1.884 |
Mainstream Media |
9 |
trump, mother, watch, family, say, try, son, kill, judge, cnn |
1610 |
0.092 |
0.515 |
1.896 |
domestic and International Conflicts |
4 |
justice, year, dead, child, ginsburg, student, life, say, attack, |
1529 |
0.088 |
0.520 |
1.884 |
Social Issues |
3 |
trump, abortion, fire, new_york, notre_dame, call, know, mayor, anti, graham |
1405 |
0.081 |
0.523 |
2.144 |
Accidents and Social News |
0 |
die, dog, last, man, spain, lose, people, |
1369 |
0.079 |
0.505 |
1.967 |
Social Issues |
6 |
trump, biden, break, case, girl, rape, |
1264 |
0.073 |
0.501 |
1.884 |
Accidents and Social News |
10 |
woman, arrest, speak, slam, beto, rivera, |
1259 |
0.072 |
0.520 |
1.853 |
Political |
8 |
first, trump, candidate, back, help, climate, |
1161 |
0.067 |
0.519 |
1.929 |
Mueller |
2 |
campaign, shock, photo, arrest, admit, game, robert_mueller, crisis, respond, complaint |
1053 |
0.06 |
0.511 |
2.031 |
Figure 10 Percentage of hyperpartisan news articles for each topic per country
5 Conclusion
Our findings provide a rich and detailed picture of the political support and media trust of visitors to hyperpartisan websites and the network structure and content of hyperpartisan news media during our study period. Differing from previous research that measured populists’ media use in cross-country surveys (Schulz, 2018), we measured visits to hyperpartisan news websites based on website visits by individuals. The use of web-tracking data for media use study has advantages over self-reported data because people tend to overestimate or underestimate their media use online (Boase and Ling, 2013). According to our study, the reach of hyperpartisan media – measured by the percentage of panelists who visited hyperpartisan news domains – is 5.32 %. Although we find a statistically significant link between support for right-wing populist parties and visits to hyperpartisan media, the association between left-wing populist party support and hyperpartisan media visits is much weaker. More importantly, perhaps, we find that distrust in public broadcasters and trust in social media both increase the likelihood of visiting hyperpartisan media websites, particularly among right-wing populist party supporters. However, among panelists who visit hyperpartisan media, we find that the attention paid to hyperpartisan media content – measured by the average time spent at hyperpartisan media domains – is not significantly associated with political attitudes variables. Factors such as website interface designs, media content categories, and website functionalities (e.g., content recommendation algorithms) might explain variances in the average amount of attention hyperpartisan consumers pay to the media content. Finally, we have been able to identify that some hyperpartisan news websites (e.g., RT and DailyKos) have transnational audiences across multiple languages, a finding that studies of national audiences might have difficulty obtaining. We believe that these findings indicate a research gap: It is critical to further investigate what is shown on hyperpartisan media websites, that is, to more deeply analyze the content of this type of media.
Our findings support previous research concerning how the professional journalism of public broadcasters can balance a polarized and partisan media environment (Sehl et al., 2020). Country comparisons suggest variances in the consumption of hyperpartisan media across the six countries studied. We found that panelists in Italy and the US, including both supporters and non-supporters of right-wing populist parties, are more likely than panelists in other countries to visit hyperpartisan media websites. According to Humprecht et al. (2020), both countries feature more polarized societies and more partisan journalism. Echoing Heft et al. (2021), we also found considerable differences in the reach of hyperpartisan websites across different countries, as in the case of some of the German hyperpartisan outlets detailed here. Next, while previous research has produced some similar findings, our research is (to our knowledge) not only the first to be based on the sources of news and hyperpartisan news but also the first to analyze the consumption of this content based on fine-grained audience data. Furthermore, we have been able to produce novel findings about these consumption patterns (such as the male and older skew of attention to hyperpartisan sites).
However, the larger context for our study is that the period under consideration was a highly volatile time in US politics, with the Mueller report into President Trump’s connections with Russia a focus of controversy, the findings of the report and resulting implications eagerly anticipated by different political factions. One obvious feature of the vast bulk of the hyperpartisan content concerning the situation was its negative and critical orientation, characterized by widespread attacks on elite progressive causes that are perceived as extreme. Meanwhile, the much less prominent left-oriented hyperpartisan sites were geared towards motivating political activists. Examples of partisan-fueled false news and conspiracy theories also feature prominently on hyperpartisan news websites. Furthermore, topic modeling results suggest that, while hyperpartisan sites in European countries disproportionately focused on Muslims and immigration, the focus in the US was on conspiracy theories and centered around party-based political attacks and attacks on leaders. This finding echoes previous studies on the country variances in online disinformation (Humprecht, 2018) . Other news content, such as reports on the Christchurch mosque shootings in March 2019 and the Notre Dame fire in April 2019, further demonstrate the global orientation of hyperpartisan websites. Another focus was US cultural and social conflicts and the fact that these were heavily concentrated in online-only (here: hyperpartisan) news media, echoing earlier findings by Neuman et al. (2014) that suggested online attention is more focused on local and social issues, with traditional (and, in that study, offline) media more focused on foreign policy and economic policy (although we should also note that our findings depart from theirs in that foreign news, especially highly controversial topics, also attract substantial attention). Furthermore, the reach of certain hyperpartisan networks and their content attract attention across national – and, seemingly, linguistic – boundaries, even if linguistic boundaries remain stronger shapers of reach and attention.
Our analysis of the content returns us to the question of what constitutes “hyperpartisanship.” That is, if news should include diverse perspectives, be objective and impartial, act as a means of incorporating various societal interests, and act as a watchdog, then clearly, even if there are inevitably blurred lines, our analysis demonstrates the analytical logic of drawing boundaries between what should count as journalism and news and what counts as news for populist supporters and falls outside of the norms of news journalism. Namely, hyperpartisan news deserves a separate category that is animated by catering to particular audiences and presenting “news” in a highly adversarial way, including attacks on what is labeled “mainstream media” or “fake news.” The absence of gatekeepers – as found in other research on populists in particular – enables challengers to question the news media and accuse them of bias.
The topics of disinformation and polarization have occupied much recent scholarship. However, our analysis points to highly selective rather than broad understandings of what should be considered news, including a focus on content with a highly conflictual understanding of certain social and cultural issues and an absence of balanced and well-supported documentation of events, sometimes extending to the embrace of conspiracy theories (recall the example of the Notre Dame-Christchurch link). In terms of the network clusters of hyperpartisan websites, beyond lone clusters such as DailyKos and foreign propaganda websites such as RT, the clusters are linguistic, and apart from in the US – where these websites supported Donald Trump’s politics – they cater to supporters of right-wing populist parties, as in the case of German websites supporting the AfD party.
Our analysis has enabled us to provide a precise way to link the supporters of certain political positions with their website visits, and to map them onto each other in a detailed manner. Although hyperpartisan websites appeal particularly to populist supporters, audiences with high levels of interest in politics also visit them disproportionately. This applies, as we have seen, to the attention given to websites such as DailyKos, a website that further exemplifies the formation of a central node in the networks of hyperpartisan websites that traverse national and linguistic boundaries. Furthermore, lower levels of trust in traditional media and higher levels of trust in social media point to the interest of populist right-wing supporters in an alternative hyperpartisan news diet. Broadly speaking, at a time of intense contention about the nature of impartiality and objectivity in news and about political partisanship, our analysis adds to this body of research the finding that, especially for right-wing populist supporters, there exists what can be called a parallel universe that has drifted away from the “mainstream” of news journalism, populated by hyperpartisan websites providing exaggerated or even falsified political information.
References
Barnidge, M., & Peacock C. (2019). A third wave of selective exposure research? The challenges posed by hyperpartisan news on social media. Media and Communication 7(3), 4 – 7.
Benkler, Y., Faris, R., & Roberts, H. (2018). Network propaganda: Manipulation, disinformation, and radicalization in American politics. Oxford University Press.
Benkler, Y., Faris, R., Roberts, H. (2017, March 3). Study: Breitbart-led right-wing media ecosystem altered broader media agenda. Columbia Journalism Review. https://www.cjr.org/analysis/breitbart-media-trump-harvard-study.php.
Bennett, W. L. and Livingston, S. (2020). The disinformation age: Politics, technology, and disruptive communication in the United States. Cambridge University Press.
Bhatt, S., Joglekar, S., & Bano, S. (2018). Illuminating an Ecosystem of Partisan Websites. In: Companion of the The Web Conference 2018 on The Web Conference 2018 – WWW ‘18, pp. 545 – 554.
Blei, D. M., Ng, A. Y., and Jordan, M. I. (2003). Latent dirichlet allocation. Journal of Machine Learning Research, 3: 993 – 1022.
Boase, J., & Ling, R. (2013). Measuring mobile phone use: Self-report versus log data. Journal of Computer-Mediated Communication, 18(4), 508 – 519.
de Vreese, C. H., Esser, F., Aalberg, T. R. (2018). Populism as an expression of political communication content and style: A new perspective. The International Journal of Press/Politics, 23(4): 423 – 438.
Evans, M.S. (2014). A computational approach to qualitative analysis in large textual datasets. Plos One 9(2): e87908.
Fawzi, N. (2019). Untrustworthy news and the media as “Enemy of the People?” How a populist worldview shapes recipients’ attitudes toward the media. The International Journal of Press/Politics, 24(2), 146 – 164.
Hallin, D. C., & Mancini, P. (2004). Comparing media systems: Three models of media and politics. Cambridge University Press.
Holt, K., Ustad Figenschou, T., & Frischlich, L. (2019). Key dimensions of alternative news media. Digital Journalism, 7(7), 860 – 869.
Howard, P. N. (2020). Lie machines: How to save democracy from troll armies, deceitful robots, junk news operations, and political operatives. Yale University Press.
Humprecht, E. (2018). Where ‘fake news’ flourishes: A comparison across four Western democracies. Information, Communication & Society, 22(13), 1973 – 1988.
Humprecht, E., Esser, F., & Van Aelst, P. (2020). Resilience to online disinformation: A framework for cross-national comparative research. The International Journal of Press/Politics 23(3): 493 – 516.
Jungherr, A. & Schroeder, R. (2021). Digital Transformations of the Public Arena, Cambridge: Cambridge University Press.
Krackhardt, D., & Stern, R. (1988). Informal networks and organizational crises: An experimental simulation. Social Psychology Quarterly, 51(2), 123 – 140.
Krämer, B. (2018). Populism, media, and the form of society. Communication Theory 28(4): 444-465.
Lewis, R. (2018). Alternative influence: Broadcasting the reactionary right on YouTube [Report]. Data & Society Research Institute.
Maier, D., Waldherr, A., & Miltner, P. (2018). Applying LDA topic modeling in communication research: Toward a valid and reliable methodology. Communication Methods and Measures, 12(2 – 3), 93 – 118.
Majó-Vázquez, S., Nielsen, R. K., & González-Bailón, S. (2018). The backbone structure of audience networks: A new approach to comparing online news consumption across countries. Political Communication, 36(2), 227 – 240.
Moffitt, B. (2017). The global rise of populism: Performance, political style, and representation. Stanford University Press.
Mourao, R. R., & Robertson, C. T. (2019). Fake news as discursive integration: An analysis of sites that publish false, misleading, hyperpartisan and sensational information. Journalism Studies, 20(14), 2077 – 2095.
Mudde, C. (2007). Populist radical right parties in Europe. Cambridge University Press.
Neuman, W. R., Lauren, G., & Jang, S. M. (2014). The dynamics of public attention: Agenda-setting theory meets big data. Journal of Communication 64(2): 193 – 214.
Newman, N., Fletcher, R., & Schulz, A. (2020). Reuters Institute Digital News Report 2020. Report.
Piantadosi, S. T. (2014). Zipf’s word frequency law in natural language: a critical review and future directions. Psychon Bull Rev, 21(5), 1112 – 1130.
Reber, U. (2018). Overcoming language barriers: Assessing the potential of machine translation and topic modeling for the comparative analysis of multilingual text corpora. Communication Methods and Measures, 13(2), 102 – 125.
Robertson, R. E., Jiang, S., & Joseph, K. (2018). Auditing partisan audience bias within google search. Proceedings of the ACM on Human-Computer Interaction, 2(CSCW), 1 – 22.
Schulz, A. (2018). Where populist citizens get the news: An investigation of news audience polarization along populist attitudes in 11 countries. Communication Monographs, 86(1), 88 – 111.
Schulz, A. (2019) Populist Citizens’ Media Perception and Media Use in Western Democracies. University of Zurich, Faculty of Arts.
Sehl, A., Simon, F. M., & Schroeder, R. (2020). The populist campaigns against European public service media: Hot air or existential threat? International Communication Gazette, 84(1). https://doi.org/10.1177/1748048520939868.
Stier, S., Kirkizh, N., Froio, C., & Schroeder, R. (2020). Populist Attitudes and Selective Exposure to Online News: A Cross-Country Analysis Combining Web Tracking and Surveys. The International Journal of Press/Politics. doi:10.1177/1940161220907018
Taneja, H., & Wu, A. X. (2019). Web infrastructures and online attention ecology. International Journal of Communication, 13, 736 – 756.
Wu, A. X., & Taneja, H. (2016). Reimagining internet geographies: A user-centric ethnological mapping of the world wide web. Journal of Computer-Mediated Communication, 21(3), 230 – 246.
Xu, W. W., Sang, Y., & Kim, C. (2020). What drives hyperpartisan news sharing: exploring the role of source, style, and content. Digital Journalism, 8(4), 486 – 505.
Yan, P., Schroeder, R., & Stier, S. (2021). Is there a link between climate change scepticism and populism? An analysis of web tracking and survey data from Europe and the US. Information, Communication & Society, 1 – 40. doi:10.1080/1369118x.2020.1864005
Acknowledgements
This research has been funded by the Volkswagen Foundation (Grant No. 94758). We are grateful to several reviewers for very helpful comments.
Supplementary Material A Summary Statistics of Web-Tracking Dataset
Figure A1 Relative percentages of panelists who visited different types of news websites in each country (N = 6,644)
Figure A2 Political orientations of hyperpartisan media domains, inferred from their visitors’ political orientations
Figure A3 Political orientations of hyperpartisan media domains compared to non-hyperpartisan media domains
Figure A4 Visualization of the number of distinct panelists (using a logarithmic axis) and average duration per visit to each hyperpartisan media domain. Number of distinct panelists is plotted in logarithmic scale with log base 10 to avoid overlaps of bubbles in the plot. Values for the number of distinct panelists in this plot could be interpreted as True value of distinct panellists= 10^(Observed value on x-axis)
Supplementary Material B Summary statistics of regression variables and analyses of visits and attention to hyperpartisan media websites
Table B1 Survey question wordings and summary statistics of independent variables in the models of visits and attention to hyperpartisan media websites
Group |
Variable |
Question wording for each variable |
Categories |
Freq (% of valid) |
Valid |
Dependent |
Visits to hyperpartisan media websites Average attention to hyperpartisan media websites |
Generated Yes based on No web tracking Min = 0 |
1192 (17.9%) 5452 (82.1%) Mean (SD): 57 (64.7) |
6,644 1,192 |
|
Political attitudes |
Support right-wing party/politician Support left-wing party/politician Left-Right Political interest Political efficacy |
Recoded based on the question: “Is there a particular political party you feel closer to than all the other parties? If yes, which one?” In politics people sometimes talk of “left” and “right.” Where would you place yourself on this scale, where 1 means the left and 11 means the right? How interested would you say you are in politics – are you ... And how much would you say that the political system in [country] allows people like you to have an influence on politics? |
Yes No Yes No 1–11 Not interested at all Somewhat uninterested Somewhat interested Highly interested 1–11 |
1,114 (16.8%) 5,530 (83.2%) 5,839 (87.9%) 805 (12.1%) Mean (SD): 5.9 (2.6) 620 (9.3%) 1,755 (26.5%) 2,903(43.8%) 1,357 (20.5%) Mean (SD): 4.5 (2.7) |
6,644 6,644 6,542 6,635 6,120 |
Media trust |
Trust in social media Trust in public Trust in private Trust in newspapers |
How much do you trust information from… 1. I trust completely 5. I don’t trust at all |
1–5 |
Mean (SD): 2.5 (1) Mean (SD): 3.1(1) Mean (SD): 2.9 (1) Mean (SD): 3.1(1) |
6,601 6,622 6,615 6,597 |
Social |
Education level Income level Age Gender Residential area Country |
What is your highest school or education qualification? Here is a list of income classes. Please tell us what class your household is in when you add up the net income of all household members: wages, pensions and other income after all taxes and social security deductions. Would you tell us what year you were born? What is your gender? Which of the following statements best applies to the residential area in which you live? |
Low education Intermediate High education Low income Intermediate income High income Male Female Metropolitan areas Non-metropolitan areas France Germany Italy Spain UK USA |
752 (11.4%) 3187(48.4%) 2639 (40.1%) 2168 (34.5%) 2310 (36.7%) 18129 (28.8%) Mean (SD): 47.5 (14.8) 3,692 (55.8%) 2,929 (44.2%) 2,572 (38.8%) 4,054 (61.2%) 1,298 (19.5%) 845 (12.7%) 1,270 (19.1%) 1,156 (17.4%) 964 (14.5%) 1,111 (16.7%) |
6,578 6,290 6,628 6,621 6,626 6,644 |
Figure B1 Visualizations of the proportion of distinct panelists who visited hyperpartisan news websites per country (left) and the distributions of average attention to hyperpartisan websites per country (right)
Table B2 Table of regression results for models of visit and attention to hyperpartisan media websites in six countries
Independent variables |
Visits to hyperpartisan media websites |
Attention to hyperpartisan media websites |
|||
B(SD) |
Odds Ratio Exp(B) |
B(SD) |
|||
Support right-wing populist party/politician Yes |
0.351*** |
(0.114) |
1.421 |
10.141*** |
(6.255) |
Support left-wing populist party/politician Yes |
0.213+** |
(0.114) |
1.237 |
-1.318*** |
(5.890) |
Left-Right |
-0.005*** |
(0.016) |
0.995 |
0.882*** |
(0.858) |
Political interest |
0.279*** |
(0.048) |
1.322 |
-0.805*** |
(2.451) |
Political efficacy |
-0.016*** |
(0.015) |
0.984 |
-0.869*** |
(0.776) |
Trust in social media |
0.134*** |
(0.040) |
1.144 |
0.539*** |
(2.021) |
Trust in public broadcasters |
-0.192*** |
(0.052) |
0.826 |
1.531*** |
(2.621) |
Trust in private broadcasters |
-0.086+** |
(0.052) |
0.918 |
-4.344*** |
(2.679) |
Trust in newspapers |
-0.023** |
(0.055) |
0.977 |
-0.863*** |
(2.856) |
Education Intermediate |
0.276*** |
(0.124) |
1.318 |
2.653*** |
(6.305) |
Education High |
0.315*** |
(0.131) |
1.371 |
5.358*** |
(6.632) |
Income Intermediate |
-0.331*** |
(0.090) |
0.718 |
0.301*** |
(4.716) |
Income High |
-0.431*** |
(0.099) |
0.650 |
-1.163*** |
(5.171) |
Age |
0.015*** |
(0.003) |
1.015 |
0.861*** |
(0.137) |
Gender Male |
0.245*** |
(0.078) |
1.278 |
-1.749*** |
(4.053) |
Region Non-metropolitan |
-0.029*** |
(0.078) |
0.972 |
6.103*** |
(4.074) |
Country Germany |
-0.168*** |
(0.145) |
0.845 |
17.845*** |
(8.234) |
Country Italy |
-0.753*** |
(0.141) |
0.471 |
-11.231*** |
(8.170) |
Country Spain |
1.453*** |
(0.113) |
4.276 |
11.037+** |
(5.904) |
Country UK |
-1.274*** |
(0.188) |
0.280 |
24.651*** |
(11.112) |
Country USA |
0.477*** |
(0.122) |
1.612 |
14.396*** |
(6.791) |
Constant |
-2.735*** |
(0.278) |
0.065 |
6.809*** |
(14.716) |
Observations |
5,593 Pseudo-R2 (McFadden) = 0.1369 Log Likelihood = --2,301.986 Akaike Inf. Crit. = 4,647.971 |
1,027 R2 = 0.083 Adjusted R2 = 0.064 Residual Std. Error = 62.148 (df = 1,005) F Statistic = 4.324*** (df = 21; 1,005) |
|||
Note: |
+p < .1, *p < .05, ** p < .01, *** p < .001 |
Table B3 Table of regression result for models of visits to hyperpartisan media websites in six countries
(showing the interaction between right-wing populist party support and country)
Dependent variable: |
||
Visit to hyperpartisan media websites |
||
Support right-wing populist party/politician Yes |
0.019*** |
(0.242) |
Country Germany |
-0.215*** |
(0.158) |
Country Italy |
-0.710*** |
(0.163) |
Country Spain |
1.392*** |
(0.119) |
Country UK |
-1.357*** |
(0.212) |
Country USA |
0.327*** |
(0.138) |
Support left-wing populist party/politician Yes |
0.190+** |
(0.114) |
Left-Right |
-0.004*** |
(0.016) |
Political interest |
0.274*** |
(0.048) |
Political efficacy |
-0.019*** |
(0.015) |
Trust in social media |
0.139*** |
(0.040) |
Trust in public broadcasters |
-0.188*** |
(0.052) |
Trust in private broadcasters |
-0.085*** |
(0.052) |
Trust in newspapers |
-0.019*** |
(0.055) |
Education Intermediate |
0.279*** |
(0.124) |
Education High |
0.309*** |
(0.131) |
Income Intermediate |
-0.325*** |
(0.090) |
Income High |
-0.433*** |
(0.099) |
Age |
0.015*** |
(0.003) |
Gender Male |
0.240*** |
(0.078) |
Region Non-metropolitan |
-0.033*** |
(0.078) |
Support right-wing populist party/politician Yes: Country Germany |
0.255*** |
(0.380) |
Support right-wing populist party/politician Yes: Country Italy |
0.043*** |
(0.334) |
Support right-wing populist party/politician Yes: Country Spain |
0.420*** |
(0.357) |
Support right-wing populist party/politician Yes: Country UK |
0.458*** |
(0.452) |
Support right-wing populist party/politician Yes: Country USA |
0.647*** |
(0.294) |
Constant |
-2.678*** |
(0.281) |
Observations |
5,593*** |
|
Log Likelihood |
-2,298.474*** |
|
Akaike Inf. Crit. |
4,650.948*** |
|
Pseudo-R2 (McFadden) |
0.1382*** |
|
Note: |
+p < .1, *p < .05, ** p < .01, *** p < .001 |
Table B4 Table of regression result for models of visits to hyperpartisan media websites in six countries
(showing the interaction between right-wing populist party support and trust in public broadcaster)
Dependent variable: |
||
Visits to hyperpartisan media websites |
||
Support right-wing populist party/politician Yes |
0.829*** |
(0.269) |
Trust in public broadcasters |
-0.153*** |
(0.055) |
Support left-wing populist party/politician Yes |
0.212+** |
(0.114) |
Left-Right |
-0.005*** |
(0.016) |
Political interest |
0.275*** |
(0.048) |
Political efficacy |
-0.017*** |
(0.015) |
Trust in social media |
0.137*** |
(0.040) |
Trust in private broadcasters |
-0.085+** |
(0.052) |
Trust in newspapers |
-0.023*** |
(0.055) |
Education Intermediate |
0.268*** |
(0.124) |
Education High |
0.305*** |
(0.131) |
Income Intermediate |
-0.337*** |
(0.090) |
Income High |
-0.433*** |
(0.099) |
Age |
0.015*** |
(0.003) |
Gender Male |
0.247*** |
(0.078) |
Region Non-metropolitan |
-0.030*** |
(0.078) |
Country Germany |
-0.195*** |
(0.146) |
Country Italy |
-0.746*** |
(0.141) |
Country Spain |
1.451*** |
(0.113) |
Country UK |
-1.281*** |
(0.188) |
Country USA |
0.470*** |
(0.122) |
Support right-wing populist party/politician Yes: trust in public braodcaster |
-0.172+** |
(0.089) |
Constant |
-2.817*** |
(0.282) |
Observations |
5,593*** |
|
Log Likelihood |
-2,300.075*** |
|
Akaike Inf. Crit. |
4,646.150*** |
|
Pseudo-R2 (McFadden) |
0.1376*** |
|
Note: |
+p < .1, *p < .05, ** p < .01, *** p < .001 |
Table B5 Table of regression result for models of visits to hyperpartisan media websites in six countries
(showing the interaction between right-wing populist party support and trust in social media)
Dependent variable: |
||
Visits to hyperpartisan media websites |
||
Support right-wing populist party/politician Yes |
-0.007*** |
(0.266) |
Trust in social media |
0.107*** |
(0.044) |
Support left-wing populist party/politician Yes |
0.223+** |
(0.114) |
Left-Right |
-0.005*** |
(0.016) |
Political interest |
0.278*** |
(0.048) |
Political efficacy |
-0.016*** |
(0.015) |
Trust in public broadcasters |
-0.193*** |
(0.052) |
Trust in private broadcasters |
-0.085+** |
(0.052) |
Trust in newspapers |
-0.026*** |
(0.055) |
Education Intermediate |
0.282*** |
(0.124) |
Education High |
0.318*** |
(0.131) |
Income Intermediate |
-0.332*** |
(0.090) |
Income High |
-0.432*** |
(0.099) |
Age |
0.015*** |
(0.003) |
Gender Male |
0.241*** |
(0.078) |
Region Non-metropolitan |
-0.026*** |
(0.078) |
Country Germany |
-0.167*** |
(0.145) |
Country Italy |
-0.764*** |
(0.141) |
Country Spain |
1.456*** |
(0.114) |
Country UK |
-1.272*** |
(0.188) |
Country USA |
0.486*** |
(0.122) |
Support right-wing populist party/politician Yes: Trust in social media |
0.138*** |
(0.092) |
Constant |
-2.656*** |
(0.283) |
Observations |
5,593*** |
|
Log Likelihood |
-2,300.849*** |
|
Akaike Inf. Crit. |
4,647.697*** |
|
Pseudo-R2 (McFadden) |
0.1373*** |
|
Note: |
+p < .1, *p < .05, ** p < .01, *** p < .001 |
Supplementary Material C Summary Statistics of
Content Analysis
Table C1 Distribution of titles in different languages in the corpus
Language |
Frequency |
Percentage |
English |
7,869 |
49.95 |
Spanish |
4,516 |
28.67 |
French |
1,789 |
11.36 |
German |
1,154 |
7.33 |
Italian |
221 |
1.40 |
Others |
205 |
1.30 |
Figure C1 Coherence scores for different numbers of topics
Date received: January 2022
Date accepted: October 2023
1 We coded right- and left-wing party/leader support in each country based on information from the PopuList (https://popu-list.org), an academic overview of populist parties in Europe. One exceptional case is the Five Star Movement (“Movimento 5 Stelle”) in Italy, which is currently coded as Euro-skeptic in the list but which we coded as a left-wing populist party for the period of early 2019.
2 https://www.diffbot.com/dev/docs/article/ and https://newspaper.readthedocs.io/en/latest/ The use of two website crawlers increases the rate of successful content crawling of news articles in the news visit dataset.
3 For example, www.conservativeinstitute.org/conservative-news/utah-judge-anti-trump.htm?utm_source=dc&utm_medium=email&utm_campaign=dc-1 Title of the page is after the last “/,” linked by “-.” It should be noted that sometimes the title is not the same as keywords in URLs, but these keywords give a relatively reliable description of the main content of the website article.
4 URLs that end with characters such as .”com,” .”com/,” .”com/#,” .”de/,”.”es/,” or .”es.”
7 We provide average duration of visits rather than overall time spent because overall time spent would be driven by extreme values (such as long time pausing within a page or accidentally leaving a page open). Average duration instead comes closer to how “sticky” a website is, which gives a better indication of the interest in a page (although all measures here are imperfect, including number of visits and overall time spent). Notably, there are only seven visitors to Politikstube, a low number compared to other site visits.
keywords
hyperpartisan media
populism
web-tracking data
computational social science
media attention
Metrics
Downloads
Published
Issue
Section
License
Copyright (c) 2024 Pu Yan, Ralph Schroeder (Author)

This work is licensed under a Creative Commons Attribution 4.0 International License.